Reasoning AI: More marketing than reality
AI agents, which are also a trend, do not yet offer the results their promoters promise.
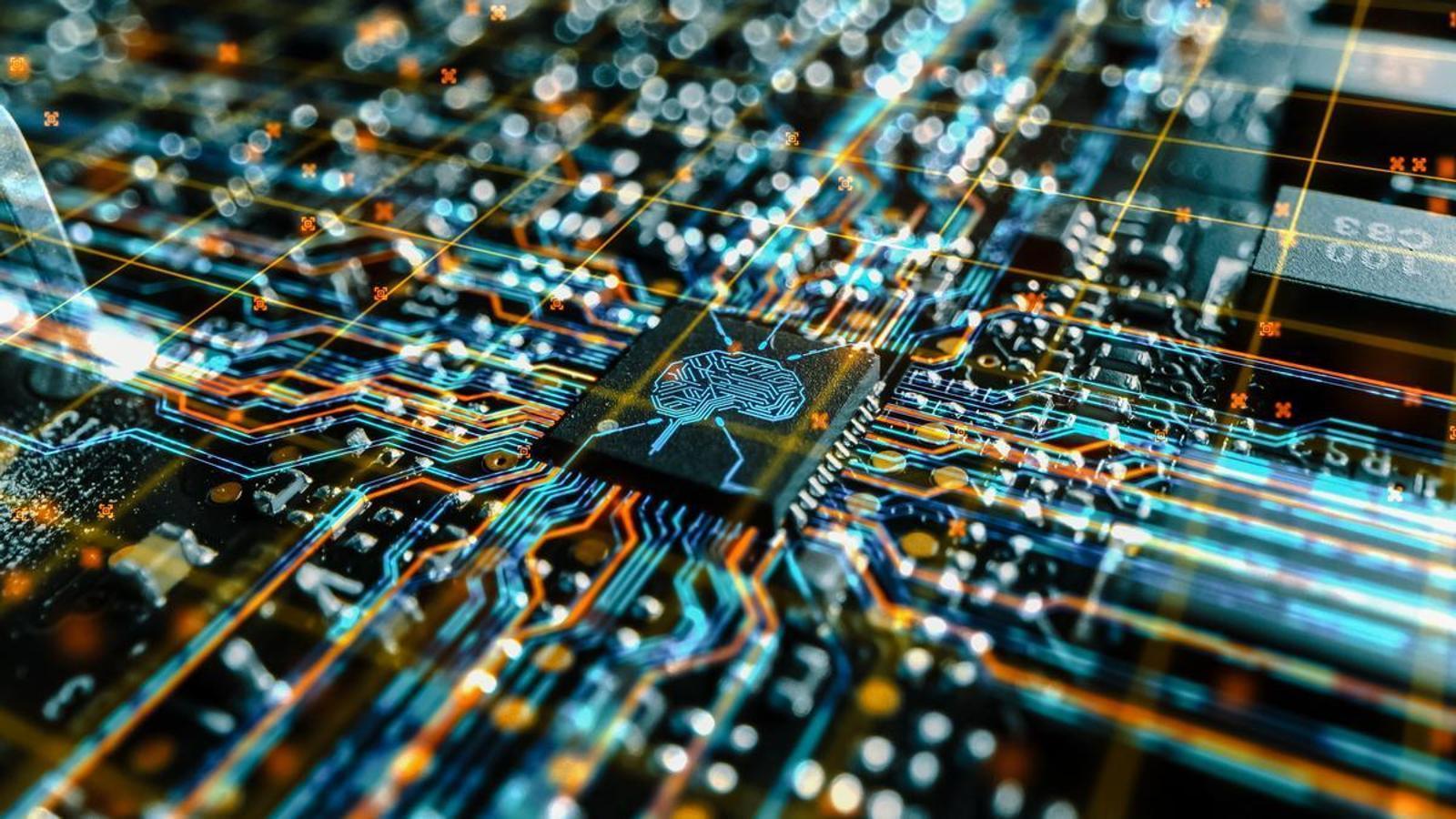
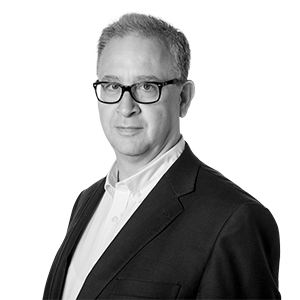
BarcelonaThe two trends that are shaping the future of applied artificial intelligence (AI) in 2025 are the so-called "deep reasoning" of chatbots and so-called "AI agents." Both promise to revolutionize our interactions with technology, but to what extent do these promises reflect reality? And how much can we trust them?
Chatbots that "reason"... or do they pretend to?
Major developers like OpenAI, Google, Anthropic, and DeepSeek claim that their most advanced models can now "reason." Unlike initial versions, which responded immediately, these new systems can spend seconds or minutes working on a problem before answering, while entertaining us by explaining what they're doing. Supposedly, this "reasoning" technology has already outperformed leading systems in often-challenged tests that measure AI progress.
But what does it really mean for an AI to "reason"? According to Dan Klein of the University of California, Berkeley, "Reasoning is when the system does extra work after being asked a question." In some cases, a reasoning system can refine its approach to a question by trying different ways of approaching it or by reviewing previous tasks. Basically, the system tries everything it can to answer.
However, researchers at Carnegie Mellon University and other institutions have put LLMs (Large Language Models) to the test on real-world tasks, such as organizing meetings, analyzing spreadsheets, or evaluating code updates. The results are not very encouraging: the best model at the time, Anthropic's Claude 3.5, only achieved a 24% success rate. Worryingly, many errors were caused by a lack of common sense or by confusing the real world with software.
A recent study by Apple also seriously questions the reasoning capabilities of current models. According to Mehrdad Farajtabar, one of the authors, he found "no evidence of formal reasoning in the language models." "Their behavior is best explained as sophisticated pattern recognition, so fragile that changing names can alter their results by approximately 10%."
Gary Marcus, a leading critic of the hype surrounding AI advances, has repeatedly pointed out that these systems systematically fail the larger the problems become. Even the most advanced models, such as OpenAI’s O1, suffer a loss of performance as task complexity grows, unlike a conventional calculator, which would maintain 100% accuracy.
A particularly revealing study by Anthropic published in October 2024, titled The biology of an LLM, examined how its own Claude 3.5 Haiku model works internally. The study revealed notable discrepancies between what the model says it does and what it actually does when processing the information. For example, when asked how it calculated 36 + 59, the model replied: "I added the units (6 + 9 = 15), carried the 1, then added the tens (3 + 5 + 1 = 9), with a result of 95." But internal analysis showed that it was actually using very different mechanisms, such as "low-precision" features to approximate the result and lookup tables to determine the final exact digit.
AI Agents: Promises Waiting for Results
If chatbot reasoning already raises questions, "AI agents"—systems designed to act autonomously on behalf of users—are even more fraught with confusion and exaggerated expectations. According to Gartner, only 6% of companies say they have implemented AI agents, even though global spending on generative AI is estimated to exceed €600 billion by the end of 2025.
“imagine the animal differently,” says Prem Natarajan, chief AI scientist at Capital One. “Many of what companies call AI agents today are really just chatbots and AI assistants,” adds Tom Coshow, an analyst at Gartner.
What makes a system truly an agent? According to Coshow, it’s defined by two simple questions: “The AI makes a decision, and the AI agent executes an action.” If those requirements aren’t met, it’s probably just another assistant.
Companies like OpenAI, Google, Microsoft, Amazon, and Anthropic are all betting big on agents, announcing new products like Amazon’s Nova Act, OpenAI’s Operator, and Computer Use by Anthropic. In business settings, it makes some sense to deploy an agent, for example, to monitor the performance of each of an operator's cell towers and apply appropriate corrections in the event of an incident, as Google unveiled this week. In contrast, in the consumer space, agents promise to automate basic tasks like ordering food or booking travel, but their reliability is very limited. Tests show that these systems are slow, struggle to operate independently for long periods of time, and make mistakes a human wouldn't make.
Privacy and security risks
The enthusiasm for these technologies often hides the significant associated risks. AI agents require deep access to consumers' digital environments, raising serious privacy concerns: they can collect a wealth of personal data, from biometric information and browsing history to financial data and purchasing patterns. Users are generally unaware of what data these agents collect, how it is used, and who has access to it.
There are also cybersecurity risks: Anthropic's experimental agent was found to have a vulnerability that could be exploited to download and execute malicious software. AI agents could be manipulated by malicious actors, who could exploit their capabilities to perform unauthorized actions or expose sensitive data. This risk is compounded by the lack of comprehensive regulatory frameworks to oversee the creation of these technologies and their implementation.
Between skepticism and commercial realities
Gartner data on investment in generative AI indicates that 80% of spending will be on hardware, such as servers, smartphones, and computers, as all manufacturers integrate AI as a standard feature in their devices, as seen at the recent MWC25. This reflects more of a strategy to force the purchase of new devices than a response to real user needs.
The reality is that, despite all the promises, neither chatbots reason how humans nor AI agents are as autonomous and capable as they would have us believe. As Gary Marcus points out, "The refuge of LLM fans is always to dismiss any individual error, but the patterns we see are too broad and systematic."
As digital giants and startups continue to tout these new capabilities—driven largely by the need to justify huge investments to shareholders—consumers and businesses alike would be wise to remain skeptical and evaluate these technologies by their tangible results, not their promises.